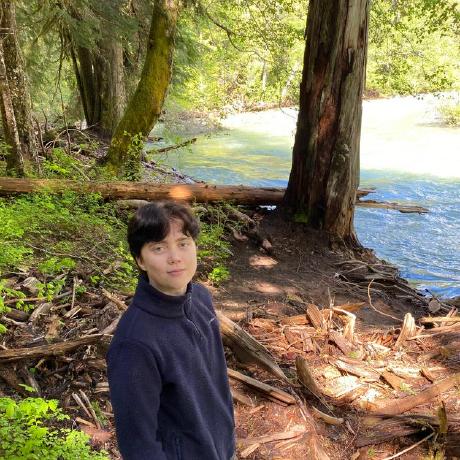
archievilliers
/
sdxl-picasso
An SDXL fine-tune based on Picasso's work
- Public
- 242 runs
- SDXL fine-tune
Want to make some of these yourself?
Run this model