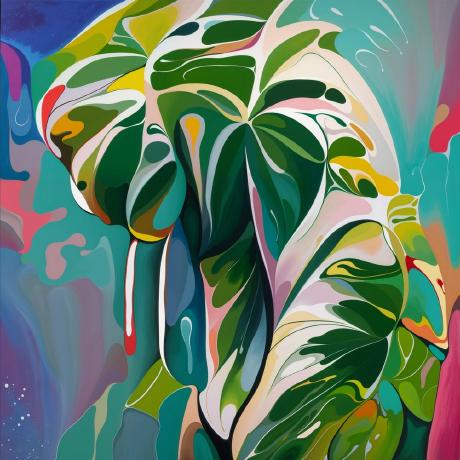
fofr
/
realvisxl-v3-multi-controlnet-lora
RealVisXl V3 with multi-controlnet, lora loading, img2img, inpainting
Want to make some of these yourself?
Run this model