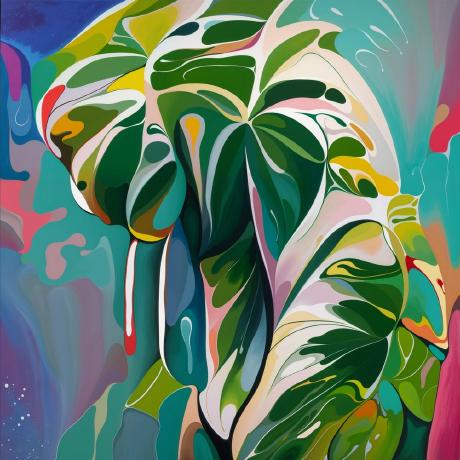
fofr
/
sdxl-multi-controlnet-lora
Multi-controlnet, lora loading, img2img, inpainting
Want to make some of these yourself?
Run this modelMulti-controlnet, lora loading, img2img, inpainting
Want to make some of these yourself?
Run this model