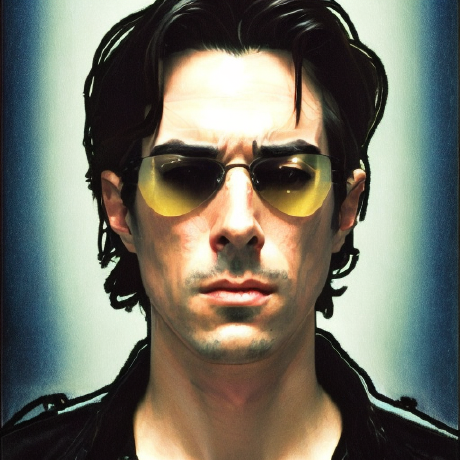
jagilley
/
controlnet-canny
Modify images using canny edge detection
Want to make some of these yourself?
Run this modelModify images using canny edge detection
Want to make some of these yourself?
Run this model