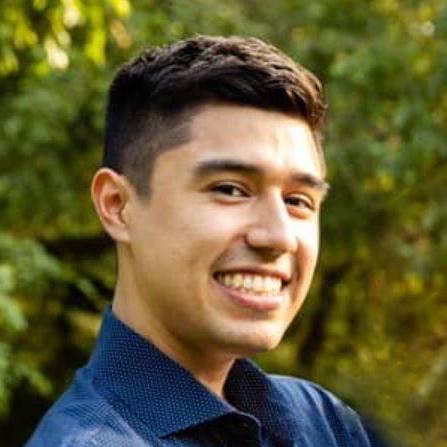
lucataco
/
flux-watercolor
A Flux LoRA trained on watercolor style photos
- Public
- 800 runs
- Fine-tune
Want to make some of these yourself?
Run this model