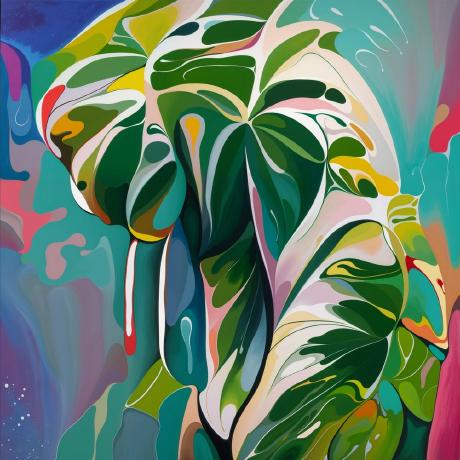
fofr
/
sdxl-barbie
A fine-tuned SDXL lora based on the Barbie movie
- Public
- 36.5K runs
Want to make some of these yourself?
Run this modelA fine-tuned SDXL lora based on the Barbie movie
Want to make some of these yourself?
Run this model