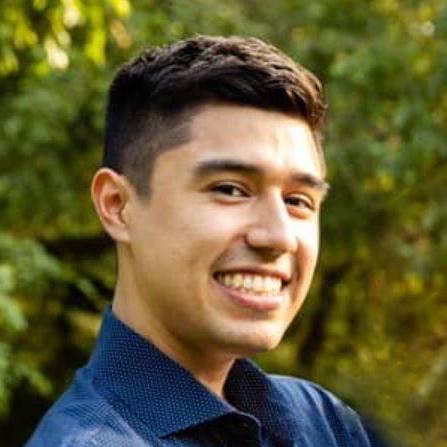
lucataco
/
diffusionlight
DiffusionLight: Light Probes by Painting a Chrome Ball
Want to make some of these yourself?
Run this modelDiffusionLight: Light Probes by Painting a Chrome Ball
Want to make some of these yourself?
Run this model